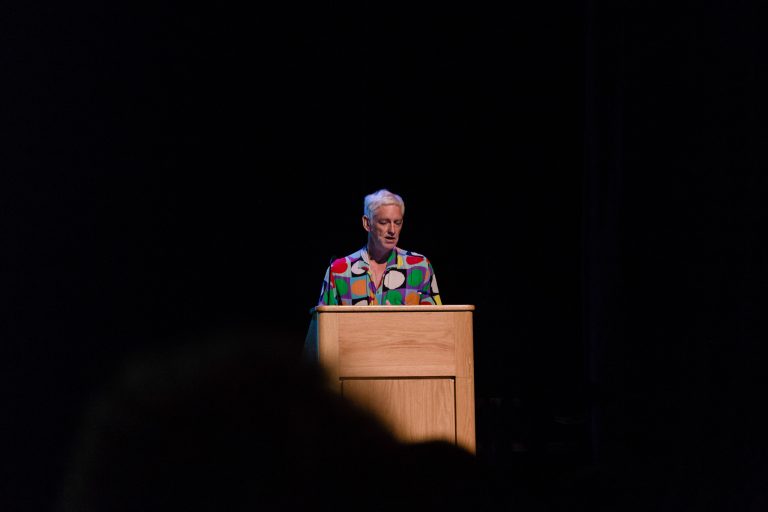
Krystal Chen
The enormous amount of data people create in the modern world is difficult to sift through. While the amount of data within our modern day society undoubtedly aids and facilitates the lives of many individuals, many questions arise as a result: how can we utilize and analyze big data efficiently and effectively? How should we derive unbiased conclusions from an avalanche of data? And through which means can we correctly interpret facts and predict outcomes from our data?
“The Distinguished Lecture Series in Data Science,” a new lecture series at UC Santa Barbara created by faculty members from the Department of Statistics and Applied Probability, offers students an opportunity to learn to understand data by applying statistical machine learning.
Peter Norvig, a director of research at Google, gave a speech in Campbell Hall regarding the importance of introducing machine learning methods to the public on Wednesday, Oct. 25. In his talk, Norvig stressed the importance of data literacy and “debuggability,” and also elaborated upon how to explain machine intelligence.
“[Our] current departments are comparatively isolated. What we need are more cross-disciplinary conversations on the base of data science.” said Sang-Yun Oh, a faculty member from UCSB’s statistics department and a co-organizer for the lecture series. His words underscore the growing demand for and reliance on data. Such demand requires current students and teaching departments to create a better technique for analyzing data — namely, the application of machine learning.
Machine learning, a data-analyzing method, is achieved through a repeated process of testing and debugging. It is a modern approach to efficiently reach a result and predict an outcome through artificial intelligence.
The series is designed to appeal to a wide audience, including UCSB students, faculty, and the general public, and offers a new vision of how data should be selected and processed. According to Oh, an accurate result has to be constructed under the efforts of three branches.
First, reasonable data is need. “Data itself can be biased and problematic,” stated Norvig in the lecture. “Machines can only learn based on data that is given,” indicated Oh during an interview with The Bottom Line.
Without background knowledge of different subjects, incorrect or irrational data can not be sorted out, which will substantially influence the analyzed outcome. In the lecture, Norvig differentiated between what individuals want, which network data implies is games and social media, and individual’s needs, such as peace and equality, to indicate the necessity of professionals to obtain effective and unbiased data.
Through continuous interviews and observations, researchers are able to eliminate these confounding variables, thus constructing an effective data base for machine intelligence.
Second, a correct statistics method is required. “Data need[s] to be processed through [an] appropriate statistic method,” Oh said. A statistical method, such as using a model-fitting technique and eliminating outliers, relates observed data to theoretical mode, thus guiding machines to provide a correct interpretation.
Third, the support of matured software is indispensable in forming machine thinking and reaching results. Current software with machine thinking replaces traditional programmers with software trainers who build machine thinking. Instead of editing code to cope with certain situation, they give computer examples, such as data and clarification, and train it to perform tasks automatically.
The examples presented by Norvig, such as how machine intelligence misinterprets pictures, were not only hilarious, but also provided the audience with a concrete idea of how participants train machines to reach results: through open-ended adjustment and testing. Thus, this matured software offers a foundation for the continued growth of machine intelligence.
On a macro level, machine thinking opens up the possibility of interpreting data and predicting outcomes. Furthermore, accurate results can be generated through the process of debugging. “Speakers of this Distinguished Lecture Series offer their view of where the data science trend is changing their institution and where it is going. I believe it is important to see the bigger picture of how the data science movement is changing some of the most influential organizations in our society and what the critical thinkers are wrestling with,” said Oh.